Data-driven Decision Making: Analytics in Digital Freight Management
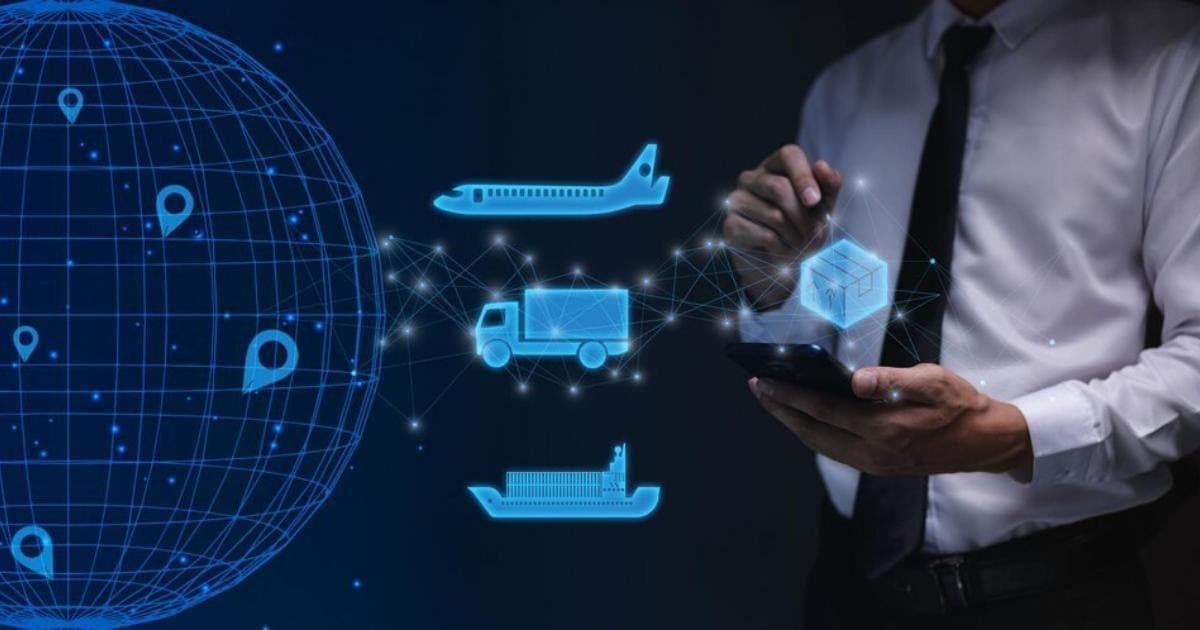
The Evolution of Freight Management
Traditionally, freight management relied heavily on manual processes and experience-based decision-making. However, with the advent of digital technologies and the exponential growth of data, the industry is undergoing a paradigm shift. Companies are now leveraging data analytics to make informed decisions, optimise operations, and enhance overall efficiency.
The Power of Data in Freight Management
Real-time Visibility:
One of the key advantages of data-driven decision-making in freight management is the ability to gain real-time visibility into the entire supply chain. Advanced tracking systems and IoT devices provide detailed insights into the location, condition, and status of shipments. This real-time data allows logistics managers to make informed decisions, proactively address potential issues, and ensure on-time deliveries.
Predictive Analytics:
Predictive analytics plays a pivotal role in optimizing freight management processes. By analyzing historical data, machine learning algorithms can forecast demand, identify potential bottlenecks, and anticipate delivery delays. This proactive approach enables companies to implement preventive measures, allocate resources efficiently, and enhance overall supply chain reliability.
Route Optimisation:
Data-driven decision-making allows for intelligent route planning and optimization. By analyzing historical traffic patterns, weather conditions, and other relevant data, logistics managers can identify the most efficient routes for transportation. This not only reduces fuel consumption and transportation costs but also minimizes delivery times, resulting in improved customer satisfaction.
Cost Reduction:
Efficiency gains from data-driven decision-making directly translate into cost savings. By identifying areas for improvement, streamlining processes, and optimizing resource allocation, companies can significantly reduce operational expenses. Whether it’s fuel costs, labor expenses, or maintenance overheads, data analytics empowers organizations to make data-driven choices that lead to a more cost-effective freight management system.
Implementing Data-Driven Decision Making
Data Integration:
The first step towards effective data-driven decision-making in freight management is ensuring seamless integration of data from various sources. This includes data from IoT devices, transportation management systems, warehouse management systems, and external sources like weather forecasts and traffic data. A centralized data repository enables a comprehensive analysis that forms the foundation for informed decision-making.
Analytics Platforms:
Investing in robust analytics platforms is crucial for deriving meaningful insights from the vast amount of data generated in freight management. These platforms utilize advanced algorithms and machine learning techniques to uncover patterns, trends, and anomalies in the data. Whether it’s identifying optimal routes, predicting maintenance requirements, or optimizing inventory levels, analytics platforms empower businesses to make data-driven decisions.
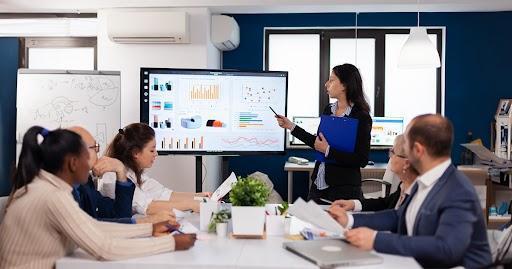
Collaboration and Communication:
Effective data-driven decision-making extends beyond analytics platforms. It requires collaboration and communication among various stakeholders in the supply chain. Sharing insights and data-driven recommendations with carriers, suppliers, and other partners fosters a collaborative approach to decision-making, resulting in a more streamlined and efficient freight management ecosystem.
Continuous Improvement:
The dynamic nature of the freight management industry necessitates a commitment to continuous improvement. Regularly reviewing performance metrics, analyzing feedback, and incorporating learnings into the decision-making process ensures that organizations stay agile and responsive to changing market conditions.
Overcoming Challenges
While the benefits of data-driven decision-making in digital freight management are evident, challenges such as data security, interoperability, and the need for skilled personnel must be addressed. Implementing robust cybersecurity measures, fostering industry-wide standards for data exchange, and investing in training programmes are essential steps to overcome these challenges and unlock the full potential of data-driven freight management.
Conclusion
In conclusion, the integration of data-driven decision-making and analytics into digital freight management is reshaping the industry landscape. The ability to harness real-time data, predict future trends, optimize routes, and reduce costs has become a competitive advantage for businesses striving to stay efficient and responsive. As technology continues to advance, embracing data-driven decision-making is not just a choice but a necessity for those looking to thrive in the rapidly evolving world of logistics and transportation.