The Role of AI and Machine Learning in Optimising Digital Freight Marketplaces
In today’s rapidly evolving logistics landscape, digital freight marketplaces are transforming the way goods are transported globally. These platforms leverage advanced technologies such as Artificial Intelligence (AI) and Machine Learning (ML) to enhance efficiency, transparency, and decision-making across the supply chain. This blog explores the pivotal role of AI and ML in optimising digital freight marketplaces, driving innovation, and reshaping logistics operations worldwide.
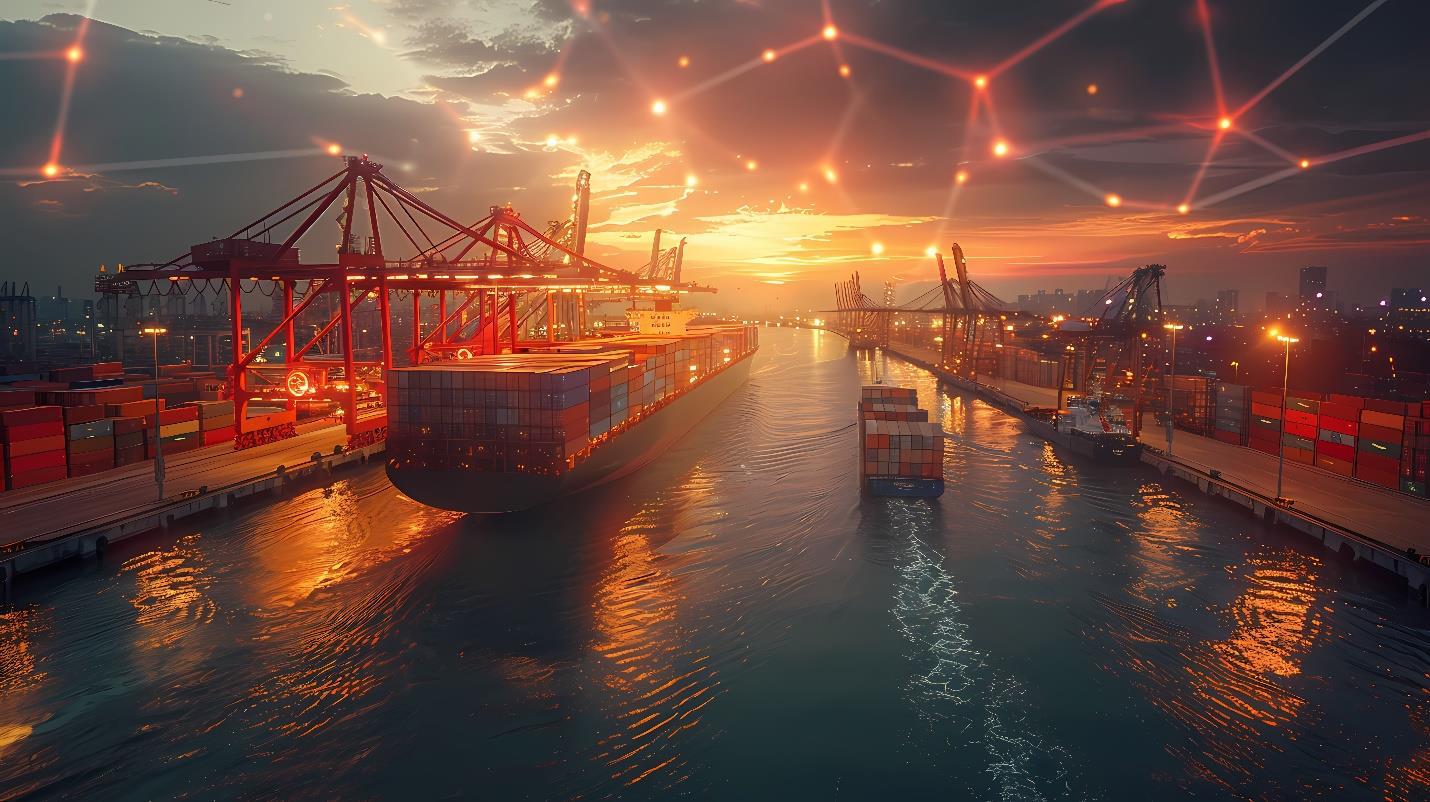
Understanding Digital Freight Marketplaces
Digital freight marketplaces act as intermediaries between shippers (companies that need goods transported) and carriers (companies that provide transportation services). Traditionally, logistics processes involved numerous intermediaries and manual tasks, leading to inefficiencies, delays, and higher costs. However, with the advent of digital freight platforms, these challenges are being mitigated through automation and data-driven insights.
The Role of AI and ML in Digital Freight Marketplaces
AI: Transforming Decision-Making
AI plays a crucial role in optimising digital freight marketplaces by leveraging vast amounts of data to make informed decisions in real-time. Here’s how AI is driving transformation:
- Route Optimisation: AI algorithms analyse historical and real-time data to suggest the most efficient routes based on factors like traffic patterns, weather conditions, and delivery deadlines. This reduces transit times and fuel consumption while optimising resource allocation.
- Predictive Analytics: By analysing historical data on shipping routes, AI can predict potential disruptions or delays. This proactive approach enables stakeholders to mitigate risks and make necessary adjustments in advance, ensuring smoother operations.
- Demand Forecasting: AI-powered algorithms analyse market trends, seasonality, and historical demand patterns to forecast future transportation needs accurately. This helps stakeholders anticipate demand surges and optimise fleet utilisation accordingly.
- Dynamic Pricing: AI enables dynamic pricing models based on real-time market conditions, demand-supply dynamics, and even environmental factors. This flexibility allows carriers to maximise revenue while offering competitive rates to shippers.
- Enhanced Customer Insights: AI algorithms analyse customer behaviour and preferences, providing valuable insights that can be used to personalise services, improve customer retention, and enhance overall satisfaction.
Machine Learning: Optimising Efficiency
Machine Learning complements AI by enabling systems to learn from data and improve over time without explicit programming. In the context of digital freight marketplaces, ML algorithms contribute significantly to efficiency and operational excellence:
- Automated Load Matching: ML algorithms match freight loads with available carriers based on criteria such as capacity, equipment type, and delivery deadlines. This automated process reduces manual effort and optimises resource allocation.
- Risk Management: ML models analyse historical and real-time data to assess risk factors associated with routes, carriers, and shipments. This proactive risk management approach helps prevent disruptions and ensures compliance with safety regulations.
- Operational Predictions: ML algorithms analyse operational data to predict maintenance needs for vehicles and equipment. By identifying potential issues in advance, logistics companies can schedule maintenance activities strategically, minimising downtime and maximising fleet efficiency.
- Natural Language Processing (NLP): NLP technologies powered by ML enable automated processing of documents, such as bills of lading and invoices. This streamlines paperwork, reduces errors, and accelerates transaction processing within the digital freight marketplace.
- Continuous Improvement: ML algorithms analyse performance metrics and user feedback to identify areas for improvement across the supply chain. This iterative process ensures that digital freight marketplaces evolve to meet changing industry demands and customer expectations.
Enhancing Operational Efficiency with Machine Learning
- Real-time Tracking and Transparency: ML-enabled tracking systems provide real-time visibility into freight movements. Shippers can monitor cargo location, temperature, and condition throughout the shipping process, ensuring compliance with regulatory standards and customer expectations.
- Automated Documentation and Compliance: Machine Learning algorithms automate paperwork processes, including customs documentation and regulatory compliance checks. This reduces administrative burdens, accelerates clearance times at border crossings, and minimises delays in shipment deliveries.
- Customer Insights and Personalised Services: ML algorithms analyse customer preferences and historical data to offer personalised shipping solutions. By understanding unique requirements, freight marketplaces can tailor service offerings, improve customer satisfaction, and foster long-term business relationships.
Case Studies: Real-World Applications
- Uber Freight: Utilises Machine Learning to match carriers with shippers based on real-time capacity and demand data. AI algorithms optimise routes and pricing, enhancing operational efficiency and reducing empty miles.
- Flexport: Integrates AI for predictive analytics, enabling shippers to anticipate supply chain disruptions and optimise inventory management. ML algorithms enhance visibility and transparency across global supply chains.
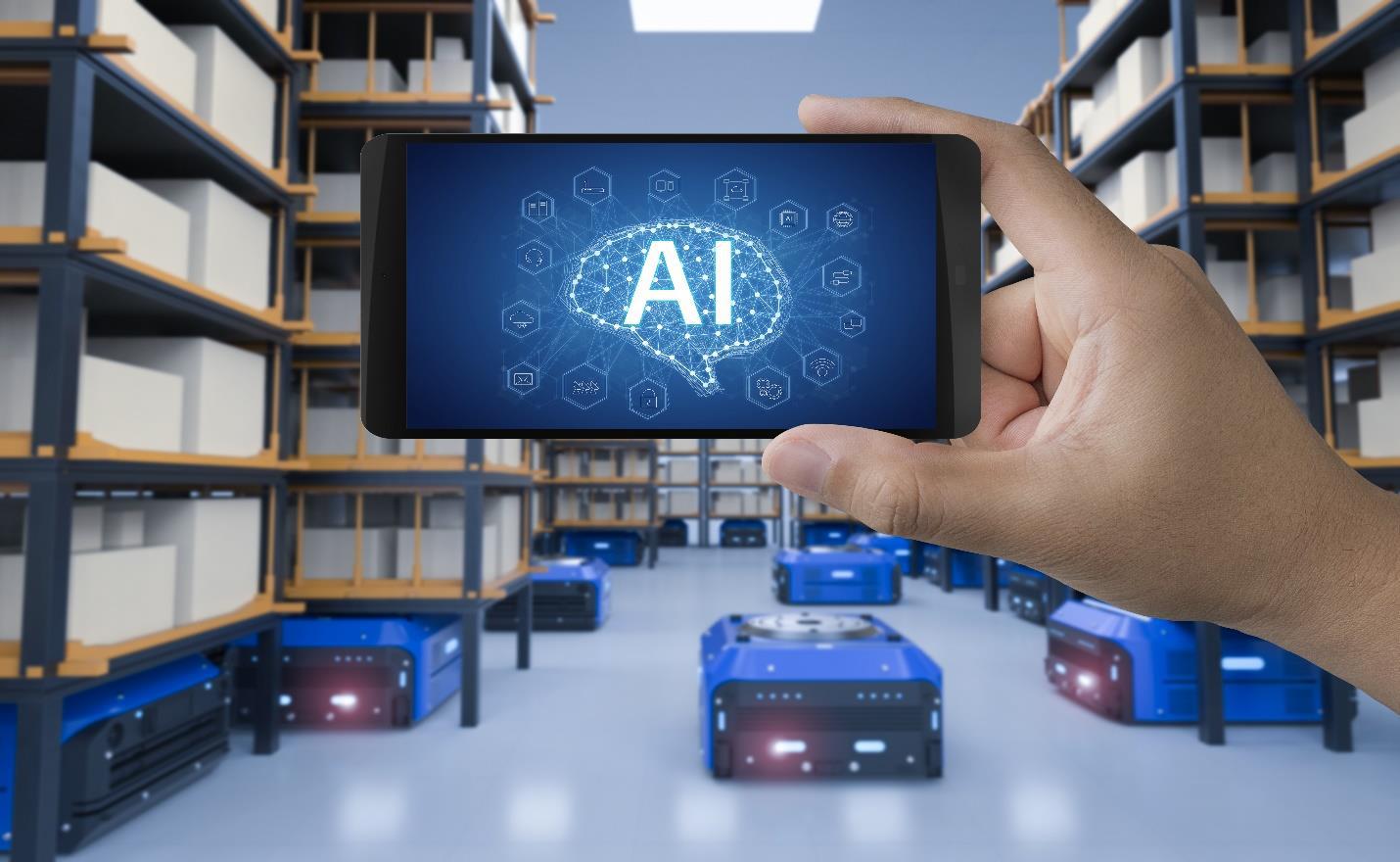
Future Trends and Innovations
- Autonomous Vehicles: AI-powered autonomous trucks and drones are poised to revolutionise freight transportation, offering faster delivery times, reduced labour costs, and improved safety.
- Blockchain Integration: Combining AI with blockchain technology enhances transparency, security, and traceability of shipments, reducing fraud and optimising supply chain visibility.
- Predictive Maintenance: ML algorithms predict equipment failures and maintenance needs in real-time, minimising downtime and ensuring fleet reliability.
- Environmental Sustainability: AI and ML algorithms facilitate eco-friendly logistics practices by optimising energy consumption, reducing carbon emissions, and promoting sustainable transportation solutions. Freight marketplaces are increasingly adopting green logistics strategies to align with global environmental regulations and consumer preferences.
Conclusion
In conclusion, AI and Machine Learning are pivotal in optimising digital freight marketplaces, driving efficiency, cost-effectiveness, and sustainability in logistics operations. As technology continues to advance, these platforms will play an increasingly critical role in reshaping the future of global supply chains. Embracing AI-powered solutions enables companies to stay competitive, enhance customer satisfaction, and navigate the complexities of modern logistics with agility and foresight.
For more insights on how AI and ML are transforming logistics and freight management, stay tuned to our blog for future updates and developments in this dynamic field.